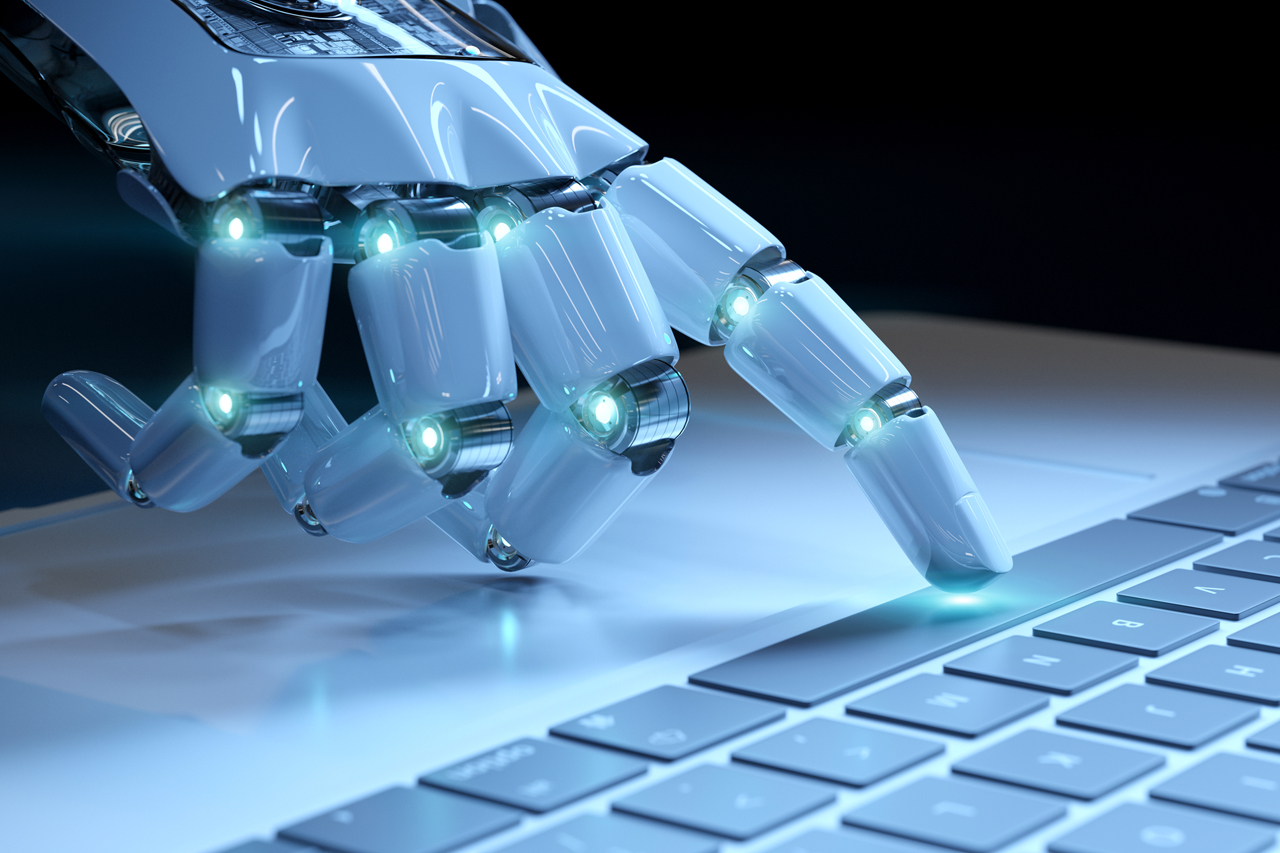
In today’s interconnected world, cybersecurity is a paramount concern for businesses, governments, and individuals alike. As technology evolves, so do the tactics of cybercriminals. Traditional methods of threat detection, while still valuable, may not be sufficient to combat the ever-growing sophistication of cyberattacks. This is where Artificial Intelligence (AI) and Machine Learning (ML) come into play. In this article, we will explore how AI and ML are revolutionizing the field of cybersecurity by providing advanced threat detection capabilities.
The Changing Landscape of Cyber Threats
The digital landscape is constantly evolving, and cyber threats have become more complex and diverse than ever before. Attackers use a variety of tactics, including malware, phishing, ransomware, and zero-day exploits, to compromise systems and steal sensitive data. Moreover, the rapid expansion of the Internet of Things (IoT) has increased the attack surface, making it more challenging to protect networks and devices.
Traditional signature-based detection methods, which rely on known patterns of malware, can be easily bypassed by new and unknown threats. Therefore, cybersecurity professionals are turning to AI and ML to enhance their threat detection capabilities.
How AI and ML Work in Threat Detection
AI and ML are subsets of computer science that enable systems to learn from data and make intelligent decisions without explicit programming. In the context of cybersecurity, these technologies are used to analyze vast amounts of data and identify patterns and anomalies that may indicate a security breach. Here’s how AI and ML contribute to advanced threat detection:
- Anomaly Detection: ML algorithms can analyze network traffic, system logs, and user behavior to establish a baseline of normal activity. When deviations from this baseline occur, it raises red flags for potential threats. For example, if a user suddenly starts accessing sensitive files they’ve never accessed before, it could indicate a security breach.
- Behavioral Analysis: AI-driven systems can continuously monitor user and device behavior. They can identify patterns that are indicative of malicious activities, such as a sudden increase in failed login attempts or unusual data transfers.
- Real-time Monitoring: AI and ML can provide real-time threat detection and response capabilities. They can detect threats as they happen and trigger automated responses, such as isolating compromised devices or blocking suspicious network traffic.
- Predictive Analysis: Machine learning models can predict potential threats based on historical data and evolving attack patterns. This proactive approach allows organizations to address vulnerabilities before they are exploited.
- Natural Language Processing (NLP): AI-powered NLP can be used to analyze and classify text-based data, such as emails and chat messages, for phishing attempts and malicious content.
- Image and File Analysis: ML algorithms can scan images and files for known malware signatures and behavioral anomalies, helping to identify malicious content that may be hidden within seemingly harmless files.
Benefits of AI and ML in Threat Detection
The integration of AI and ML into cybersecurity practices offers several significant advantages:
- Speed and Scalability: AI-driven threat detection systems can process large volumes of data in real-time, making them suitable for monitoring complex and high-traffic environments.
- Adaptability: ML algorithms can continuously learn and adapt to new threats without human intervention. This flexibility is crucial in combating evolving cyber threats.
- Reduced False Positives: ML algorithms are designed to minimize false positives by focusing on unusual patterns and behaviors, reducing the burden on security teams to investigate benign alerts.
- Automation: AI and ML can automate many aspects of threat detection and response, allowing security teams to focus their efforts on more complex tasks.
- Early Detection: Machine learning models can identify subtle signs of a breach that may go unnoticed by traditional methods, enabling organizations to respond before significant damage occurs.
Challenges and Considerations
While AI and ML offer promising capabilities in threat detection, there are challenges and considerations to keep in mind:
- Data Privacy: The analysis of large datasets for threat detection raises concerns about data privacy and compliance with regulations such as GDPR and HIPAA. Organizations must implement robust data protection measures.
- False Negatives: While ML can reduce false positives, it’s not immune to false negatives. Threats that exhibit unusual but not obviously malicious behavior may go undetected.
- Training Data: ML models require extensive training data to be effective. Ensuring the quality and diversity of training data is essential to avoid biases and inaccuracies.
- Cybersecurity Skills Gap: Implementing AI and ML in cybersecurity requires skilled professionals who understand both the technology and the threat landscape. The cybersecurity skills gap remains a challenge.
- Adversarial Attacks: Attackers are increasingly using adversarial techniques to deceive AI and ML systems. This requires ongoing research and development to stay ahead of adversaries.
Real-World Applications
AI and ML are being used across various industries to enhance threat detection:
- Financial Services: Banks and financial institutions use ML to detect fraudulent transactions in real-time by analyzing transaction patterns and user behavior.
- Healthcare: ML is employed to identify abnormal access to patient records, protecting sensitive healthcare data from unauthorized access.
- E-commerce: Online retailers use AI-powered fraud detection systems to identify and prevent fraudulent transactions and account takeovers.
- Critical Infrastructure: Industries like energy and utilities rely on AI and ML to monitor and protect critical infrastructure against cyber threats.
- Cloud Security: Cloud service providers use AI and ML to monitor and protect their platforms, identifying and mitigating threats across vast cloud ecosystems.
The Future of Advanced Threat Detection
As the cybersecurity landscape continues to evolve, AI and ML will play an increasingly critical role in advanced threat detection. These technologies have the potential to stay ahead of cybercriminals by continuously learning and adapting to new threats. However, it’s important to remember that they are not a silver bullet and should be used in conjunction with traditional security measures, such as firewalls and antivirus software.
The key to effective threat detection lies in a multi-layered approach that combines human expertise with AI and ML capabilities. Cybersecurity professionals will need to develop their skills in data science and AI to harness the full potential of these technologies. In an era where cyber threats are constantly evolving, leveraging AI and ML for advanced threat detection is not just a choice but a necessity to protect our digital assets and data.